Berkeley Students Help You Squat with a Digital Personal Trainer
Due to the COVID-19 shelter-in-place guidelines, many gym-goers have shifted their workout routines to their homes. However, without personal trainers, many of these gym-goers are struggling to do their exercises, particularly squats, correctly and are subject to injuries. As an initiative to solve this, a group of five UC Berkeley students have used cutting edge machine learning tools to create the Squat Assistant. The Squat Assistant is designed to help users to adjust their squatting position so that they can squat correctly and get the most out of their workout by providing a simple way to monitor themselves independently by just using their cameras.
The Squat Assistant takes in the user’s webcam feed, maps key joints of the user’s body into sets of coordinates, and then uses a neural network to classify if the squat is good or bad. The students taught the algorithm how to identify good and bad squats using squat videos sourced from Youtube. The five team members used innovation engineering practices such as inductive learning and entrepreneurial behavior in order to finish the project within just one semester.
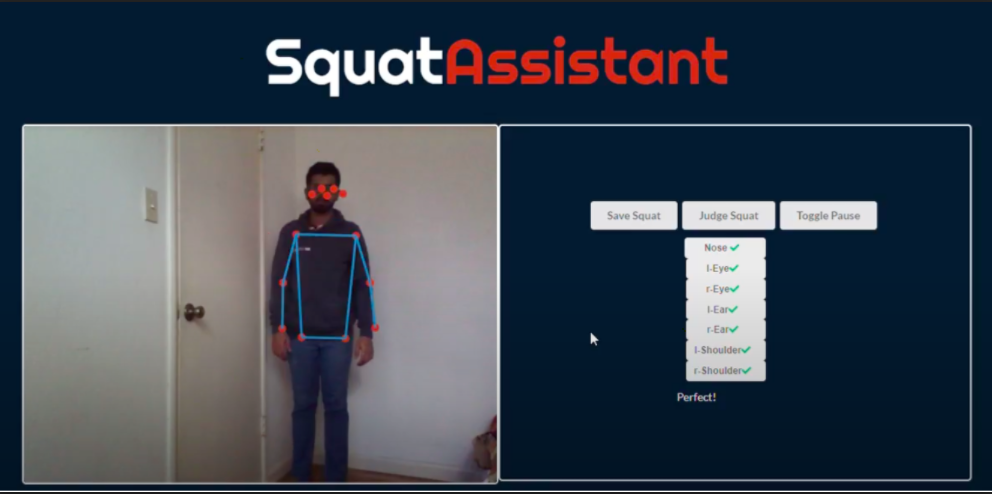
They were challenged to work with advanced technologies such as real-time human pose estimation as well as faced difficulties implementing a UI and integrating the backend with the front end. Not only did the team develop the technology, but treated the product as an entrepreneurial venture and went through industry-like innovation engineering practices such as inductive learning, agile product development, and team growth. They were able to brainstorm and pivot their initial complex ideas into a simple product concept for the fitness market. The team intends to add more features in the future and bring the production version of their app to the market.
Project by: Francho Achacoso, Meher Arora, Suhas B Umesh, Kimberly Chua, Kathy Weng Ka Leng