Materiall Brings Personalized Home Recommendations
Computer Vision brought to the art of home buying.
Berkeley, CA, November 2020 — Searching for homes can be a drag, especially when you have a vision of what you are looking for in a home. You could be searching on Realtor or Zillow for hours scrolling through hundreds of pictures just to see if the kitchen has the exact granite countertop you want. A team of UC Berkeley students have teamed up with a local start-up, Materiall, and the students have recently created a number of models that will aid Materiall’s goal of giving home-buyers a much more personalized experience when searching for a home. This comes at a critical time, when in-person home viewing has stalled and the online real estate market has taken a much bigger role in the art of homebuying. The classifier that UC Berkeley students created consists of two models, one that differentiates pictures of the inside or outside of a home, and another model that assigns labels to the rooms of the inside pictures. The models will be harnessed onto Materiall’s recommendation system where they will play a vital role in finding specific features and add to the usual filters that are currently on popular real estate websites like Zillow or Realtor.

Above is an image from the ADE20K scene parcer, and what images look like when they are ran through the models created by the UC Berkeley students. In this picture, different items in the kitchen are segmented. Using computer vision, the last step is to label the different items in the image. This is great news for both the home buyer and home seller, since this recommendation system will speed up the process of home buying: the seller sells the house faster and the home buyer can find the home of their dreams with ease. On current real estate listing websites, there are currently only filters like number of bedrooms, number of bathrooms or year built. With these breakthroughs in computer vision and the ability to label features within the rooms, a vast number of filters is now achievable.
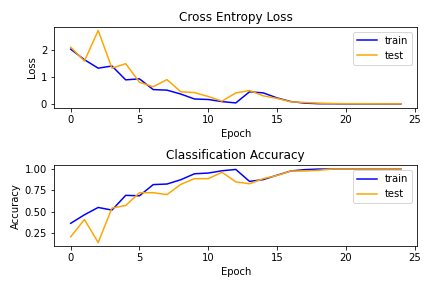
Focusing on the models, it was of utmost importance that the model built by the student team inputted an image and outputted the correct room label. With this, a significant portion of the recommendation model would be ready. Achieving this feat was no easy task, but as noted in the figure above, the model achieved near 100% accuracy. With these models, the next step is to implement a scene parser that will segment various parts of the images and classify all the different elements in a room, say an island in a kitchen or a fireplace in a living room. By combining elements of data science and computer vision, the amount of time it will take to find your dream home will be considerably faster.
About Us
The team consists of Daniel del Carpio (Data Science), Samantha Tang (Data Science), Vincent Lao (Data Science and Statistics), Parker Nelson (Data Science) and Bharadwaj Swaminathan (Data Science and Economics). All of the members on the team have data science internship experience, varying from data analysis, machine learning and data engineering. Notably, Samantha’s previous experience with AWS allowed her to spearhead the storage of the images on their remote server, and Parker’s start-up experience gave him the skills to be the product manager and delegate operations within the team.
Contact Information
Daniel Alejandro del Carpio Samantha Tang
Vincent Lao Parker Nelson
Bharadawaj Swaminathan Bharat Vijay
Github Repository: https://github.com/ddelcarpio/materiall-image-classification-fa20