NExtNet Knowledge Graph: A Data-Driven Pipeline to Optimize Drug Discovery
With the outbreak of COVID-19, the standardized process for researching, developing, and testing a drug (vaccine in this case) has taken center stage. Developing an effective drug is both costly and time-consuming. Looking specifically at cancer drugs, it is estimated that the median cost of bringing a new cancer drug to market is over $2 Billion. Copious resources are spent on R&D and understanding how past clinical trials should inform current efforts at drug development.
To tackle this problem, a group of 4 UC Berkeley students has taken a data-driven approach to understand past research and its relevance to drug discovery. Working alongside NExtNet, an AI-powered immunotherapy startup, the group has developed a data-centric pipeline to focus on identifying clinically relevant cell-based immunotherapy targets to treat many types of cancer.
A cancer researcher would be able to make use of the pipeline by viewing and interacting with a visual representation of connections found across many academic papers, called a knowledge graph (left image). The aim is to understand the relationships between these sources of information and extract the most relevant ones to validate proper treatments (right image is an example relationship).
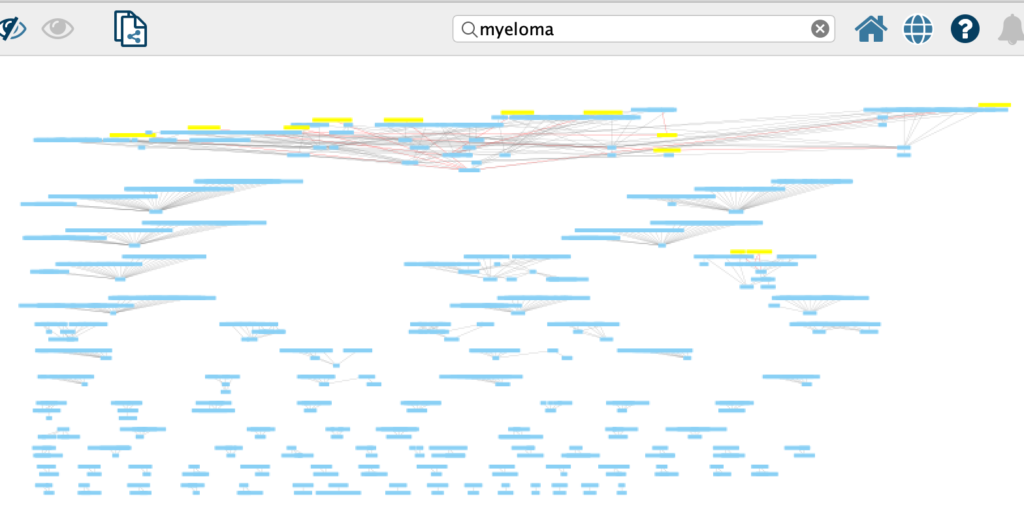
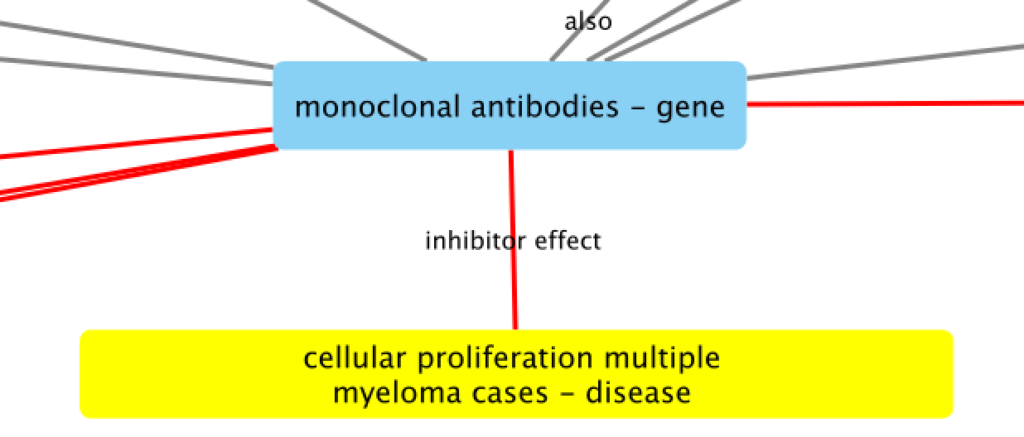
Compared to the traditional method of manually going through hundreds of past papers, finding key trends through the knowledge graph will change the complete landscape of drug discovery. Not only does this new method deliver a more accurate, detailed picture for drug development, but it also allows the potential for future pharmaceuticals to create personalized therapies for every single cancer patient with data at its core.
Find their code at this GitHub Repo.
1 https://www.biospace.com/article/median-cost-of-bringing-a-new-drug-to-market-985-million/#:~:text=A%20study%20published%20by%20the,time%20costs%20of%20%241.2%20billion